User:CJut/sandbox
Telemedicine[edit]
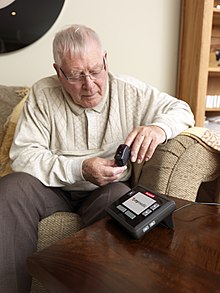
The increase of telemedicine, the treatment of patients remotely, has shown the rise of possible AI applications.[1] AI can assist in caring for patients remotely by monitoring their information through sensors.[2] A wearable device may allow for constant monitoring of a patient and the ability to notice changes that may be less distinguishable by humans. The information can be compared to other data that has already been collected using artificial intelligence algorithms that alert physicians if there are any issues to be aware of.[2]
Since the average age has risen due to a longer life expectancy, artificial intelligence could be useful in helping take care of older populations.[3] Tools such as environment and personal sensors can identify a person’s regular activities and alert a caretaker if a behavior or a measured vital is abnormal.[3] Although the technology is useful, there are also discussions about limitations of monitoring in order to respect a person’s privacy since there are technologies that are designed to map out home layouts and detect human interactions.[3]
Electronic health records[edit]
Electronic health records (EHR) are crucial to the digitalization and information spread of the healthcare industry. Now that around 80% of medical practices use EHR, the next step is to use artificial intelligence to interpret the records and provide new information to physicians.[4] One application uses natural language processing (NLP) to make more succinct reports that limit the variation between medical terms by matching similar medical terms.[4] For example, the term heart attack and myocardial infarction mean the same things, but physicians may use one over the over based on personal preferences.[4] NLP algorithms consolidate these differences so that larger datasets can be analyzed.[4] Another use of NLP identifies phrases that are redundant due to repetition in a physician’s notes and keeps the relevant information to make it easier to read.[4]
Beyond making content edits to an EHR, there are AI algorithms that evaluate an individual patient’s record and predict a risk for a disease based on their previous information and family history.[5] One general algorithm is a rule-based system that makes decisions similarly to how humans use flow charts.[6] This system takes in large amounts of data and creates a set of rules that connect specific observations to concluded diagnoses.[6] Thus, the algorithm can take in a new patient’s data and try to predict the likeliness that they will have a certain condition or disease.[6] Since the algorithms can evaluate a patient’s information based off of collective data, they can find any outstanding issues to bring to a physician’s attention and save time.[5] One study conducted by the Centerstone research institute found that predictive modeling of EHR data has achieved 70–72% accuracy in predicting individualized treatment response.[7] These methods are helpful due to the fact that the amount of online health records doubles every five years.[8] Physicians do not have the bandwidth to process all this data manually, and AI can leverage this data to assist physicians in treating their patients.[8]
Bibliography[edit]
- ^ Hamet, Pavel; Tremblay, Johanne (2017-04-01). "Artificial intelligence in medicine". Metabolism. Insights Into the Future of Medicine: Technologies, Concepts, and Integration. 69: S36–S40. doi:10.1016/j.metabol.2017.01.011. ISSN 0026-0495.
- ^ a b Pivovarov, Rimma; Elhadad, Noémie (2015-09-01). "Automated methods for the summarization of electronic health records". Journal of the American Medical Informatics Association. 22 (5): 938–947. doi:10.1093/jamia/ocv032. ISSN 1067-5027.
- ^ a b c Pouke, Matti; Häkkilä, Jonna (2013-12-12). "Elderly Healthcare Monitoring Using an Avatar-Based 3D Virtual Environment". International Journal of Environmental Research and Public Health. 10 (12): 7283–7298. doi:10.3390/ijerph10127283.
{{cite journal}}
: CS1 maint: unflagged free DOI (link) - ^ a b c d e Ferrante, Frank E. (June 2005). "Evolving Telemedicine/eHealth Technology". Telemedicine and e-Health. 11 (3): 370–383. doi:10.1089/tmj.2005.11.370. ISSN 1530-5627.
- ^ a b Medrano, Ignacio Hernández; Guijarro, Jorge Tello; Belda, Cristóbal; Ureña, Alberto; Salcedo, Ignacio; Anke, Luis Espinosa; Saggion, Horacio (2018). "Savana: Re-using Electronic Health Records with Artificial Intelligence". IJIMAI. 4 (7): 8–12. ISSN 1989-1660.
- ^ a b c Eren, Ali; Subasi, Abdulhamit; Coskun, Osman (2008-02-01). "A Decision Support System for Telemedicine Through the Mobile Telecommunications Platform". Journal of Medical Systems. 32 (1): 31–35. doi:10.1007/s10916-007-9104-x. ISSN 1573-689X.
- ^ Bennett, Casey C.; Doub, Thomas W.; Selove, Rebecca (April 2012). "EHRs connect research and practice: Where predictive modeling, artificial intelligence, and clinical decision support intersect". Health Policy and Technology. 1 (2): 105–114. doi:10.1016/j.hlpt.2012.03.001. ISSN 2211-8837.
- ^ a b Hernandez Medrano, Ignacio; Tello Guijarro, Jorge; Belda, Cristobal; Urena, Alberto; Salcedo, Ignacio; Espinosa-Anke, Luis; Saggion, Horacio (2018). "Savana: Re-using Electronic Health Records with Artificial Intelligence". International Journal of Interactive Multimedia and Artificial Intelligence. 4 (7): 8. doi:10.9781/ijimai.2017.03.001. ISSN 1989-1660.